TrustworthyAI
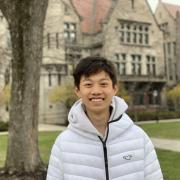
The rapid advancements in computer vision technology have led to an increase in interest in developing custom image recognition models for diverse applications. This includes the implementation of said computer vision technology into self-driving vehicles. As we implement more and more technology into our daily lives, we want to be able to comfortably use the advantages of computer vision while keeping our safety in mind. TrustworthyAI refers to the concept of developing and deploying artificial intelligence systems in a way that ensures they are reliable, ethical, transparent, and safe for both users and society as a whole. Our main focus is on the safety of self-driving cars by recognizing hazards like vandalized stop signs or cyber attacks intended to disrupt the AI. In our pursuit of achieving Trustworthy AI through computer vision, we undertook a comprehensive exploration of diverse methodologies to enhance the performance of machine learning models. Our approach involved assembling datasets comprising of both pristine and "cyber-attacked" stop signs. Following meticulous collection, we subjected the datasets to various augmentation and preprocessing techniques to introduce variability. Employing state-of-the-art models, including YOLOv8 and YOLOv5, each implementation featuring distinct convolutional neural networks and single-shot multi-box detectors, we endeavored to create multiple model versions. The primary objective was to systematically modify aspects of the dataset with each iteration, aiming to unravel the factors crucial for improving the model's discernment between a regular stop sign and one subjected to a cyber attack. Initial iterations, such as Model Version 1, exhibited a commendable confidence level in identifying stop signs. However, these versions struggled to discriminate stop signs from other objects within the images. Subsequent iterations (Model versions 2 to 5) showed incremental improvements but fell short of meeting our stringent criteria. Remarkably, Model version 6, incorporating YOLOv8, emerged as the standout performer. This iteration demonstrated an impressive accuracy of 95% in correctly identifying a normal stop sign. We established a critical threshold of 95% confidence for normal stop signs, designating anything below this level as susceptible to potential cyberattacks. Examining the results across versions, we found that lower confidence levels for normal stop signs corresponded to higher confidence in detecting cyber attacks. Notably, our base images, initially registering 0% recognition for a normal stop sign, exhibited an 89% confidence level in identifying a simulated cyber attack. This underscores the model's adaptability and capacity to recognize anomalies, even in scenarios where standard stop sign recognition might be compromised. Analyzing specific model versions provided deeper insights into performance variations across diverse datasets. Version 5 demonstrated consistently high confidence levels (95% to 97%) in recognizing normal stop signs, highlighting the model's reliability. Conversely, Version 2 encountered challenges in stop sign recognition, particularly in Image 2, where the confidence level dropped to 0%. The success of Model Version 6 underscores the potential of advanced computer vision models in enhancing safety for autonomous vehicles. These results pave the way for further advancements in Trustworthy AI, providing valuable insights for the ongoing development of ethical and reliable AI systems in real-world applications.