Latency SLOs Done Right
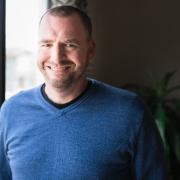
Median, average, 90th, 99th percentile. We've all seen these metrics on dashboards, both open source and from commercial vendors. And chances are that if you have used these metrics to set service level objectives or service level indicators, you've gotten it wrong. Maybe you've done something as innocuous as averaging percentiles. Or something more subtle, such as choosing histogram bin boundaries that obscure important system modes.
Today in operational time series data analysis, percentiles have become one of the primary service level indicators for representing real systems monitoring performance. When used correctly, they provide a robust metric that can be used for base of mission critical service level objectives. However, they have subtle limitations that are not infrequently overlooked by implementers.
Latency SLOs are somewhat delicate to implement, especially across clusters of nodes. This session will show three different approaches to correctly calculating latency SLOs, and how histograms and Python can be used to calculate mathematically correct quantiles and set SLOs based on those. Attendees should have experience with web services backed by multi-node clusters, and preferably have used at least one different monitoring visualization system that works with percentiles.